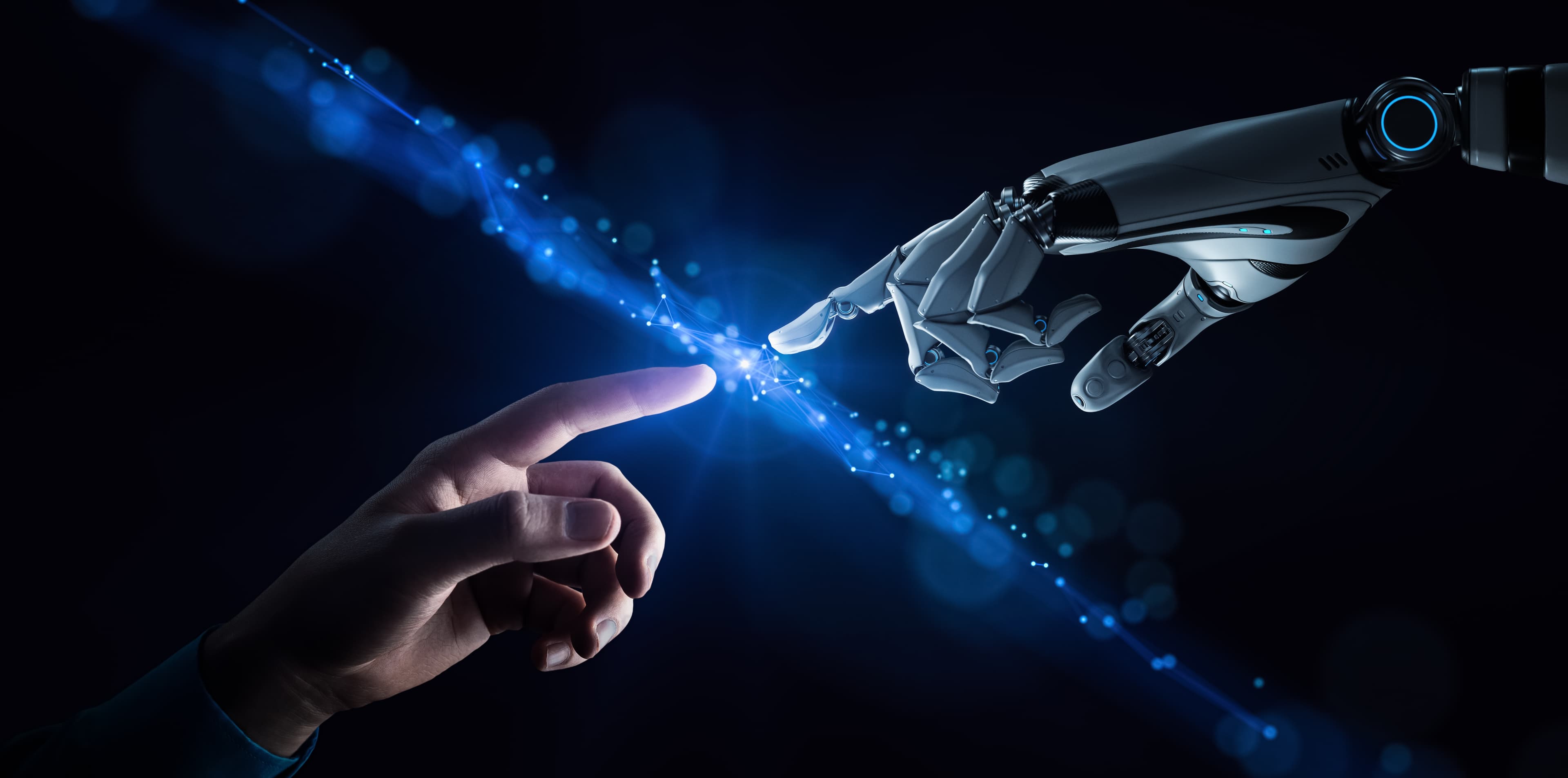
Who’s in the Loop: AI or Humans?
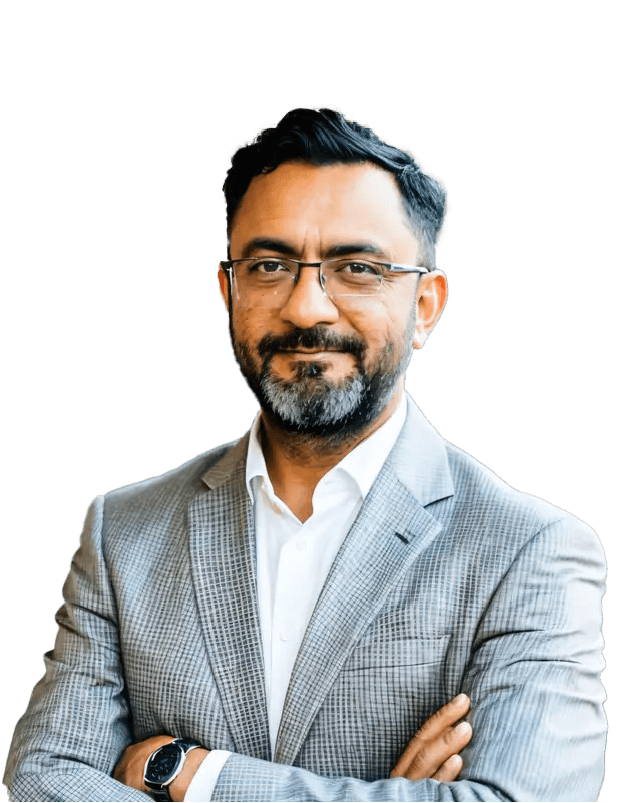
Co-Founder & CTO, Cyware
RSAC 2025 delivered big with unforgettable keynotes, packed halls, groundbreaking innovations, and conversations that pushed the boundaries of what is next in cybersecurity. And now, as the spotlight shifts to GISEC, the momentum continues. If there is one topic stealing the show across both events, it is Agentic AI. It is not just trending, it is the most urgent and transformative conversation happening in cybersecurity today, and I have no doubt you are seeing the same.
While heading to Dubai this week, flipping through the schedule and side panels, one question kept surfacing: Who should be in the loop? Humans or AI? This isn't merely philosophical, it's operational, strategic, and existential. As we build AI agents into our workflows, the way we define their agency will separate leaders from laggards. Organizations need to move beyond asking what AI can do to how we shape its role. Is your AI just a co-pilot, or is it flying the plane with humans in the jump seat?
Clearly, the path forward isn’t binary. It’s about designing the right loop for the right moment. Let machines scale and let humans apply judgment where it matters most. Agentic AI won’t succeed based on technology alone. Success will depend on how we shape the relationship between humans and machines.
This isn’t about replacing analysts; it’s about rethinking how they work, alongside agents that don’t just assist but actively drive decisions.
Now for the twist. The terms “human in the loop” and “AI in the loop” may seem straightforward, but the real question is: Who leads? Who acts? Who makes the decisions? This goes beyond semantics; it’s a structural issue that matters.
To understand this, we need to start with a foundational concept: agency. It’s not just about what an AI agent is capable of, but what it is allowed, trusted, and expected to do within a system. Agency is about intent like the difference between an automated script and a decision making entity. As we integrate AI into cyber defense, intelligence, and automation, understanding this nuance is critical.
Understanding Agency in AI Systems
In simple terms, agency refers to the ability to make independent choices and take actions that influence one's environment and having control and autonomy to shape outcomes.
What is an Agency for AI?
In AI context, agency means a system can act independently on behalf of users or organizations without constant human intervention. This makes AI truly agent-like, distinguishing it from simple tools that merely wait for commands. AI with agency makes informed decisions and adapts to changing circumstances to achieve goals, similar to how analysts operate based on experience and situational context. AI with agency is distinguished by several defining traits:
- Independent Operations: AI with agency can perform tasks autonomously, without constant human input, allowing for efficient execution and human focus on higher-level decisions.
- Purpose-Driven Actions: These AI systems are goal-oriented, proactively working toward specific outcomes, not just responding to commands.
- Environmental Adaptability: AI agents can sense and adapt to changes in their environment, adjusting their actions based on situational awareness.
- Informed Decision-Making: Agency enables AI to evaluate data, assess risks, and make decisions based on predefined criteria to achieve goals.
- Continuous Evolution: AI with agency learns from experience and feedback, improving over time to stay effective and relevant in a changing landscape.
In cybersecurity, the agency enables AI to function autonomously across several critical functions. AI agents can monitor network traffic in real time, identifying anomalies without explicit instructions. They can assess threat severity and organizational impact, prioritizing incidents that demand immediate attention. Beyond detection, AI can trigger preliminary response actions for known threats, using predefined playbooks to mitigate damage before human intervention.
More advanced systems can adapt to emerging threats, adjusting their parameters based on new intelligence and proactively seeking out indicators of compromise linked to evolving attack vectors. Throughout these processes, AI agents document their actions and reasoning, maintaining an audit trail for human analysts to review and verify.
The level of agency granted to an AI system is a pivotal design choice, influencing the entire security operation.
This brings us to the next crucial topic: loop models, which define where control and decision-making authority lie.
AI-in-the-Loop Model
What is the AI-in-the-Loop Model?
The AI-in-the-Loop model may seem counterintuitive at first. Despite its name, this approach places humans at the center of decision-making, with AI acting as an augmentation tool. In this model, human analysts remain in control and responsible for intelligence operations, while AI systems act as advanced assistants that enhance human capabilities.
How Does the AI-in-the-Loop Model Work?
In practice, AI supports humans throughout the process by handling data processing tasks: gathering and normalizing threat data, identifying patterns within large datasets, and generating initial assessments based on historical data.
Human analysts then review the insights generated by AI, using their contextual knowledge, strategic understanding, and ethical judgment to make final decisions. Analysts retain the authority to accept, adjust, or reject the AI's suggestions, considering factors the AI may not fully grasp, such as organizational risk tolerance, business context, or geopolitical nuances.
The Significance of AI-in-the-Loop in Machine Learning
From a machine learning perspective, this model provides several advantages. By keeping humans intimately involved in the decision process, the system creates continuous feedback loops that improve the AI's performance over time. Each human intervention effectively becomes a training opportunity, helping the AI better align with analyst expectations and organizational priorities.
This approach also addresses a common challenge in cybersecurity machine learning: the problem of novel threats. AI systems trained on historical data may struggle with zero-day attacks or unprecedented techniques, but human oversight ensures these blind spots don't lead to critical security failures.
Examples of AI-in-the-Loop in Cyber Threat Intelligence
- In threat hunting scenarios, an AI system might flag unusual network behavior based on statistical anomalies, but human analysts determine whether these anomalies represent genuine threats or benign business activities.
- In vulnerability management, AI tools might prioritize vulnerabilities based on technical severity and exploit availability, but human experts adjust these priorities based on the business criticality of affected systems and compensating controls already in place.
- During incident response, AI assistants can rapidly correlate alerts across security tools to identify attack patterns, while human analysts make the ultimate determination about response actions based on business impact considerations and strategic knowledge.
The Concept of Agency in this Model
Within the AI-in-the-Loop framework, AI agency remains deliberately constrained. The system possesses limited autonomy to perform well-defined tasks and make preliminary assessments, but lacks the authority to make final determinations or execute significant actions independently. The AI serves primarily as an intelligent assistant rather than an autonomous decision-maker.
Benefits of the AI-in-the-Loop Model
This approach offers several distinct advantages for cybersecurity operations.
- Human oversight in critical security decisions: Reduces the risk of automated systems making costly mistakes or being manipulated by adversaries.
- Eases adoption: Allows organizations to gradually increase AI involvement as trust develops, rather than requiring a complete paradigm shift.
- Combines human and AI strengths: Leverages human strengths in contextual understanding, ethical reasoning, and novel thinking alongside AI's advantages in processing speed, consistency, and pattern recognition, creating a powerful partnership where each component compensates for the limitations of the other.
Human-in-the-Loop Model
What is the Human-in-the-Loop Model?
The Human-in-the-Loop model represents a more AI-centric approach where autonomous systems handle most routine operations independently, with humans serving primarily as supervisors and exception handlers.
How Does the Human-in-the-Loop Model Work?
In this model, AI systems operate with significantly greater autonomy, handling common scenarios end-to-end. The AI continuously monitors environments, makes determinations, and executes actions without requiring human approval for each step.
Human analysts enter the process only at specific juncture, when the AI's confidence falls below predetermined thresholds, when conflicting indicators create ambiguity, or when the situation falls outside the AI's authorized parameters. The human acts as a "failsafe" to handle exceptions rather than as the primary driver of the process.
The Significance of Human-in-the-Loop in Machine Learning
This model represents a more advanced stage of AI implementation, typically achieved after systems have demonstrated reliable performance under AI-in-the-Loop conditions. From a machine learning perspective, it allows for greater operational efficiency while maintaining a mechanism for continuous improvement.
When humans intervene in exceptional cases, these interventions create valuable training data for expanding the AI's capabilities. Over time, this approach can systematically reduce the frequency of human intervention by converting previously exceptional scenarios into part of the AI's standard repertoire.
Examples of Human-in-the-Loop in Cyber Threat Intelligence
- Autonomous data collection and processing: The platform autonomously gathers, processes, and correlates threat data from numerous sources, reducing manual effort for routine updates.
- Automated intelligence report generation: It generates and distributes intelligence reports tailored to different stakeholders, automating the process without human oversight for regular updates.
- Alert triage and escalation: The system independently investigates low-risk alerts and dismisses common false positives, escalating only when it detects significant threats or unfamiliar patterns.
- Containment actions for known threats: For known malware families, the platform follows predefined playbooks to automatically contain the threat, only involving humans for novel variants or when business-critical systems might be affected.
The Concept of Agency in this Model
This approach grants AI systems substantially greater agency, with broader autonomy to operate independently within their defined domains. The AI possesses decision-making authority for routine matters and can execute actions with real-world consequences without requiring case-by-case approval.
However, this agency remains bounded by carefully defined parameters. The system recognizes its own limitations and knows when to seek human input, creating a safety mechanism that prevents unchecked autonomous operation in high-risk scenarios.
Benefits of the Human-in-the-Loop Model
- Improved operational efficiency: AI handles routine tasks independently, allowing human analysts to focus on complex problems, strategy, and creative responses to emerging threats.
- Scalable security operations: With AI taking over repetitive tasks, organizations can scale their security efforts without the need to proportionally increase headcount.
- Faster response times: The model speeds up threat mitigation by removing the bottleneck of human review for common and low-risk threats.
Which Approach is Best for Your Organization?
The choice between the AI-in-the-Loop and Human-in-the-Loop models depends on your organization’s maturity and needs.
- For organizations just starting with AI, the AI-in-the-Loop model is the best option. It allows teams to build confidence in AI while maintaining control over security decisions and automating low-risk tasks.
- As your Cyber Threat Intelligence (CTI) function matures, you can gradually transition to the Human-in-the-Loop model for more complex tasks. This shift should begin with low-risk activities and expand to higher-risk areas as trust in AI grows.
- Most organizations will benefit from a hybrid approach, where routine tasks are automated, but high-impact decisions and novel threats still involve human oversight. The key is to balance AI and human capabilities to optimize cybersecurity operations effectively.
How to Choose the Right Model for Your Organization
Here are some of the key factors to consider when selecting the most effective AI integration model for your organization’s cybersecurity needs, balancing automation and human expertise.
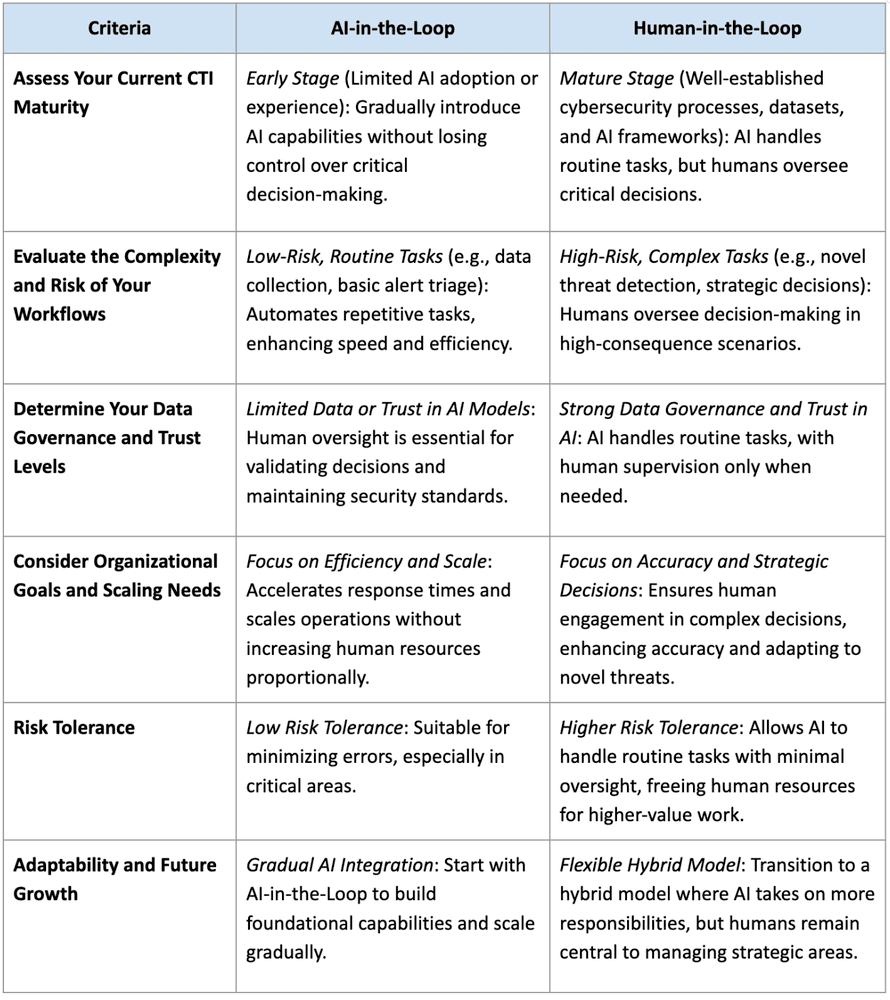
Choosing the right AI model for your organization
Conclusion
The most successful implementations of AI in cybersecurity focus on optimizing the collaboration between human expertise and AI capabilities rather than maximizing AI autonomy. By aligning your choice with your organization's security maturity, risk tolerance, and operational needs, you can unlock AI's transformative potential while maintaining appropriate oversight.
To learn more about how Agentic AI can elevate your security operations, feel free to reach out to the Cyware team.