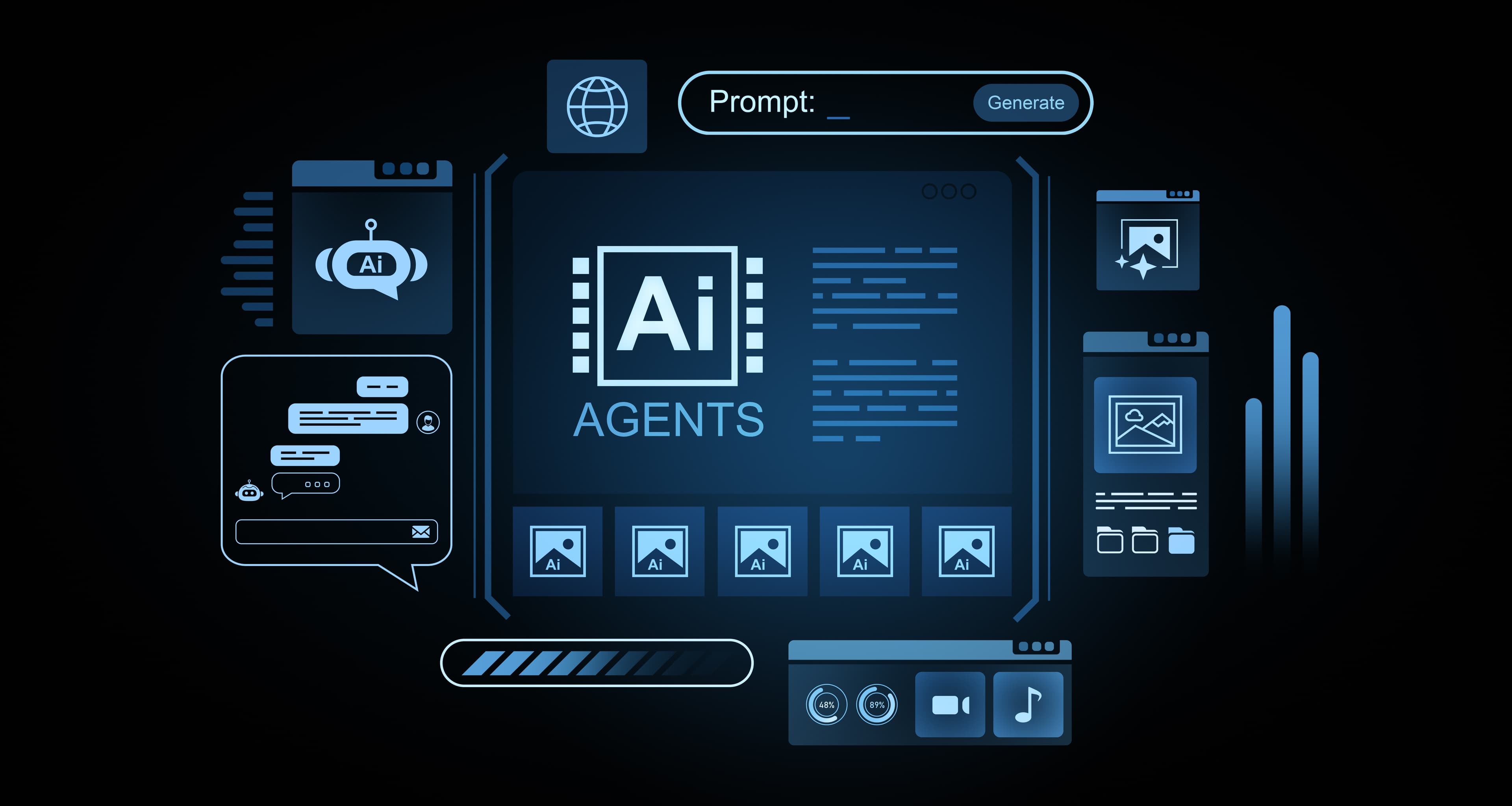
Activating AI Agents: Building a Smarter Cyber Threat Intelligence System
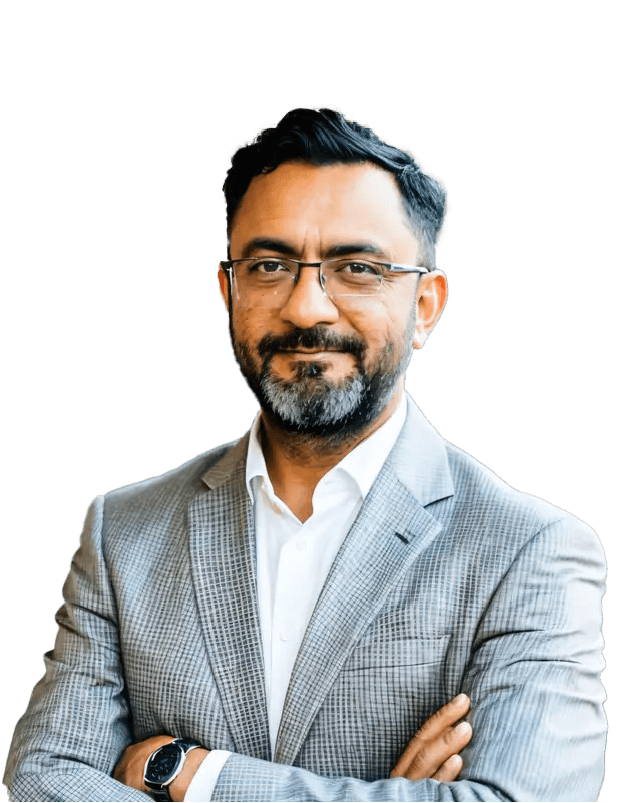
Co-Founder & CTO, Cyware
AI agents and the excitement surrounding them are more than justified. These intelligent systems are no longer just futuristic concepts; they are rapidly evolving and actively transforming industries with unparalleled speed and precision. A recent Gartner report highlights that, by 2028, 33% of enterprise software applications will include agentic AI, with at least 15% of daily operational decisions being made autonomously through AI agents.
In my last blog, I explored how AI agents represent the next major leap in security automation. Unlike traditional AI assistants that simply respond to queries, AI agents go further- they analyze, act, and automate responses in real time. While the idea of AI agents handling every possible task at lightning speed is exciting, the reality is that AI agents are still in their early stages of evolution.
Right now, the focus should be on strategic application i.e. leveraging AI agents where they can deliver immediate and transformative results with minimal to no risk to security operations.
To determine the most valuable use cases, organizations must address three critical aspects:
- Prioritizing High-Impact Use Cases: Identify the most time-intensive challenges faced by the Cyber Threat Intelligence (CTI) teams and determine which areas require immediate intervention.
- Evaluating Risk and Uncertainty: Assess the potential risks and limitations of AI agents in threat intelligence workflows, ensuring that automation enhances security without introducing vulnerabilities.
- Implementing AI with Precision: Deploy AI agents in low-risk, high-value areas where they can deliver tangible improvements in efficiency and decision-making.
By focusing on high-impact, low-risk applications, organizations can build a strong foundation for AI adoption—enhancing threat intelligence, automation, and decision-making while ensuring security operations remain resilient.
The Toughest Challenges for Cyber Threat Intelligence Teams
CTI teams are on the front lines of identifying, analyzing, and mitigating threats before they escalate into attacks. However, with threats growing more sophisticated and attack surfaces expanding, these teams must navigate an increasingly complex landscape- often with limited resources. This creates significant challenges that impact their effectiveness, including:
- Information Overload: Analysts are inundated with thousands of daily indicators of compromise (IOCs), threat bulletins, and vulnerability disclosures. CTI teams spend most of their time sifting through data, including false positives and noise, directly impacting time-to-detection.
- Lack of Context: Raw threat data often lacks relevance, leading to alert fatigue and misprioritized threats. For instance, a banking trojan IOC in a manufacturing network diverts attention from real industry-specific threats.
- Time-Sensitive Analysis: Threat intelligence has a short shelf life, yet manual processes delay response times. With breaches rising- 78% of organizations reported being breached at least once in 2024, up from 63% in 2022- faster, more proactive intelligence analysis and operationalization is essential.
- Siloed Intelligence: Threat data is often scattered across multiple tools and teams, preventing a unified, real-time view of the threat landscape. This fragmentation weakens correlation efforts and slows response times.
- Resource Constraints: The cybersecurity skills gap leaves CTI teams understaffed while threats continue to rise. Nearly 62% of organizations report shortages in their cybersecurity workforce, making it difficult to scale intelligence operations.
By leveraging AI-driven automation and AI agents, CTI teams can boost efficiency, improve threat visibility, and accelerate response times. However, AI adoption comes with challenges, as AI agents are still evolving and maturing.
While AI has the potential to enhance threat intelligence efforts, it is not a plug-and-play solution as of now.
Challenges Surrounding AI Agents That Warrant Caution
While AI agents are transforming cybersecurity with enhanced efficiency, accuracy, and speed, their responsible implementation requires addressing several strategic considerations:
- Over-Reliance on AI: While AI can streamline threat detection, excessive dependence without human oversight can create blind spots, making it harder to identify novel or sophisticated threats that don't match historical patterns.
- Lack of Explainability: Many AI models function as "black boxes," making it difficult for security teams to interpret and justify AI-driven decisions. This transparency gap impacts governance and accountability, especially in regulated industries where decision traceability is mandatory.
- Adversarial Attacks: Attackers can manipulate AI models with adversarial inputs, tricking them into misclassifying threats or bypassing security defenses.
- Data Privacy Risks: AI-driven security tools process vast amounts of sensitive data, making them high-value targets for cybercriminals. Protecting this data is essential for security and compliance.
- Model Poisoning: Attackers can corrupt AI training data, altering model behavior to bypass security measures or facilitate unauthorized access.
Beyond these security risks, operational and strategic challenges also need attention. Biases, outdated data, and coverage gaps can impact AI accuracy, necessitating continuous refinement. A hybrid approach—where AI augments human expertise rather than replaces it—is critical for effective threat response. Strong governance is also essential, ensuring well-defined roles, compliance measures, and accountability in AI-driven security operations. Despite these challenges, advancements in AI governance, continuous model training, and structured human-AI collaboration are helping organizations harness AI's full potential.
With the right safeguards in place, AI agents can drive smarter, faster, and more proactive threat intelligence capabilities.
The Sweet Spot for AI Agents in CTI: High-Impact Use Cases
AI agents' true power in cybersecurity comes from their ability to handle structured, repeatable, and data-intensive tasks with unprecedented precision and speed. When strategically deployed in the right areas, their benefits far outweigh the risks.
Below are six key use cases where AI delivers immediate value, operating within well-defined guardrails to enhance security operations without governance concerns.
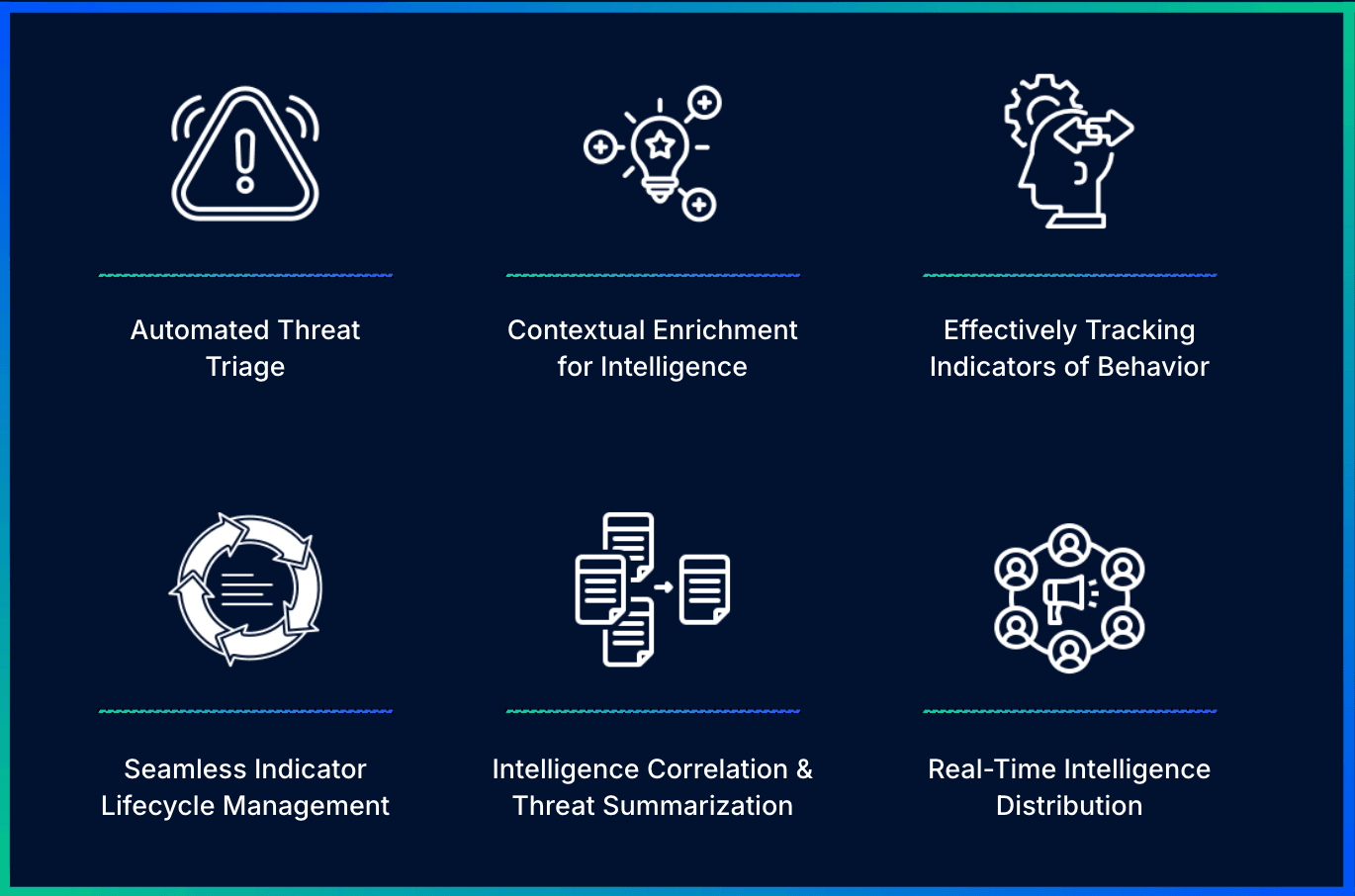
- AUTOMATED THREAT TRIAGE
The current challenge: CTI teams are inundated with thousands of threat indicators daily —ranging from IP addresses and file hashes to phishing domains and vulnerability disclosures. Sorting through this flood of data manually is inefficient and often leads to alert fatigue and missed threats.
How AI Agents can help: AI agents continuously scan incoming intelligence feeds, categorizing and prioritizing threats based on factors like threat relevance, severity, historical context, and attack patterns. By leveraging machine learning and rule-based processing, AI can automatically filter out false positives and surface high-priority threats.
Why Automation is Low-Risk and Highly Effective: Threat triage follows predictable patterns, making it an ideal candidate for AI-driven automation. AI agents can apply consistent logic, eliminating bias and fatigue that often affect human analysis. The process operates within clearly defined parameters where AI excels at pattern recognition and statistical analysis while leaving critical decision-making to security professionals.
- CONTEXTUAL ENRICHMENT FOR INTELLIGENCE
The current challenge: Raw threat indicators lack the context needed for effective response. Understanding whether a particular threat actor targets your industry or whether a vulnerability exists in your technology stack requires cross-referencing multiple data sources—a time-consuming process.
How AI Agents can help: AI can automatically enrich threat data by correlating indicators across disparate sources, adding crucial context like threat actor profiles, affected technologies, historical attack patterns, and potential impact scenarios. This enrichment transforms isolated data points into actionable intelligence tailored to your organization's specific risk profile.
Why Automation is Low-Risk and Highly Effective: Intelligence enrichment relies on systematic data correlation and pattern matching—exactly what AI algorithms excel at. AI isn't making security decisions; it's enhancing context and depth. The process follows clear rules for data augmentation and integration, ensuring automation without compromising data integrity or analysis quality. AI agents can instantly cross-reference multiple datasets, a task that would take humans hours.
- EFFECTIVELY TRACKING INDICATORS OF BEHAVIOR (IOBS)
The current challenge: IoBs are dynamic tactics and techniques adversaries use during an attack. These behaviors are subtle, often evolve over time, and are scattered across logs and telemetry. Manually identifying and linking these behaviors to malicious activity requires deep expertise, significant time, and cross-referencing of multiple data points.
How AI Agents can help: AI agents can autonomously analyze and correlate behavioral signals across the kill chain by continuously monitoring system activity, logs, and patterns of behavior. They can map behaviors to adversary playbooks, hi
ghlight likely threat actors or affected assets, and flag deviations from normal baselines revealing the attacker's true intent and not just the siloed facts at surface level.
Why Automation is Low-Risk and Highly Effective: AI agents focus on correlation and context rather than enforcement, supporting analysts without introducing operational risk. By learning from threat models and adapting behavioral baselines, they effectively surface stealthy and unknown threats- which is a great addition to IOCs.
- SEAMLESS INDICATOR LIFECYCLE MANAGEMENT
The current challenge: Threat intelligence has a short shelf life—adversaries constantly shift tactics, making yesterday's indicators irrelevant today. Manually managing thousands of indicators leads to outdated blocklists, wasted investigation time, and false positives that weaken security.
How AI Agents can help: AI agents continuously monitor threat indicator lifecycles—automatically deprecating stale indicators, tracking evolving adversary infrastructure, and identifying recurring threat patterns. By automating indicator hygiene, AI helps security teams focus on relevant threats, minimizing wasted effort and enhancing accuracy.
Why Automation is Low-Risk and Highly Effective: AI isn't adding or removing indicators arbitrarily—it follows defined rules and data-driven insights. Analysts can still review and approve changes, keeping full control over security actions. AI can track expiration timelines, observe infrastructure changes, and detect behavioral shifts—tasks that are repetitive but critical for maintaining effective threat intelligence.
- INTELLIGENCE CORRELATION AND THREAT SUMMARIZATION
The current challenge: Security teams are bombarded with vast amounts of raw threat intelligence scattered across multiple tools (SIEMs, TIPs, EDRs, firewalls, etc.). Manually summarizing, correlating, and extracting key insights is time-consuming, prone to inconsistencies, and delays critical security decisions. Analysts must stitch together fragmented data to identify broader attack campaigns—an error-prone process that can allow threats to slip through undetected.
How AI Agents can help: AI agents autonomously correlate intelligence across platforms, identifying relationships between seemingly unrelated alerts and distilling vast amounts of data into concise, actionable insights. For example, AI can detect that a phishing email campaign is linked to an IP address flagged in firewall logs, revealing a coordinated attack attempt. By surfacing hidden connections and summarizing intelligence into key takeaways, AI enables security teams to quickly assess threats and take proactive measures.
Why It's Easy to Automate With No Risk: AI isn’t generating new intelligence—it’s enhancing visibility by connecting existing data and summarizing it efficiently. Security teams retain control by validating AI-driven insights before taking action. Since AI excels at pattern recognition and data processing, it can rapidly analyze millions of data points, uncovering critical intelligence that human analysts might overlook—without introducing risk.
- REAL-TIME INTELLIGENCE DISTRIBUTION
The current challenge: Security teams struggle to rapidly distribute intelligence to the right tools and teams. Manual reporting delays critical threat sharing, slowing response times for SOC analysts, endpoint teams, and key stakeholders.
How AI Agents can help: AI agents automate intelligence distribution by pushing IOCs to security tools (firewalls, EDRs, SOAR playbooks), generating SOC incident tickets, and creating tailored reports for different audiences. They can deliver technical insights to operations teams, impact summaries to executives, and targeted advisories to business units—ensuring the right information reaches the right people without adding to analysts' workload.
Why It's Easy to Automate With No Risk: Intelligence distribution follows structured templates ideal for automation. The process uses clear logic—AI determines who needs what information and delivers it instantly based on predefined rules. AI isn't interpreting intelligence—it's distributing validated intelligence efficiently through established channels while allowing human analysts to add critical insights when needed.
Conclusion
The transformative potential of AI agents in cyber threat intelligence is undeniable. By strategically implementing the high-impact use cases outlined above, CTI teams can achieve immediate operational gains while establishing the governance foundation needed for more advanced applications.
Organizations that thoughtfully integrate AI agents into their intelligence workflows, targeting specific pain points with appropriate guardrails, will outperform those rushing to automate everything at once. The effectiveness of AI agents depends not on their raw capabilities, but on our deployment strategy.
The near-term future belongs to a symbiotic relationship between human analysts and AI systems—combining machine speed with human insight to create intelligence capabilities neither could achieve alone, gradually evolving from AI-in-the-loop to human-in-the-loop models as trust and capabilities mature.
Stay tuned for the next installment in this "AI Agents for Security Operations" series!